A lot of people can quote the common mantra of "Rubbish In Rubbish Out" and understand that getting data correct, of the right quality is a must but how many do pay attention to it? Most organizations have automated data collection processes thus data is readily available but does anyone pay attention to how it is collected?
There are many aspects to data management, for instance governance, quality, lineage, storage and processing. Getting it useful for analytics, data science or artificial intelligence is a big challenge on its own. Here I am going to share a few examples. I hope my readers (and my ardent supporters), be equipped to share the importance of getting the data correct before doing any data science project lest the insights may contain biases that can be disastrous to the business.
Example 1: Abraham Wald
This example happened in World War II. During this period, a very influential group made up of mathematicians and statisticians was set up in the United States called Statistical Research Group, at Columbia University. Why are they influential? Well, they study data and worked out mathematical equations to give the US Army the mathematical advantage during the War. In the Group, there is a very clever mathematician called Abraham Wald.
One of the problems that SRG had to work on was how to minimize bomber losses to enemy fire. Bombers when they go through enemy terrain braved a lot of enemy fire. Their bodies are huge and thus it is unlikely they are not shot at all. To protect and increase the likelihood of the pilots, together with the bomber comes back to base, SRG will like to determine which part of the bomber should be strengthened with more armor. During wartime, resources are scarce and a fully armored bomber will make it heavy and reduce its maneuverability. So they collected data from bombers that came back from the mission, look at where the bullet holes are. Below is the picture.
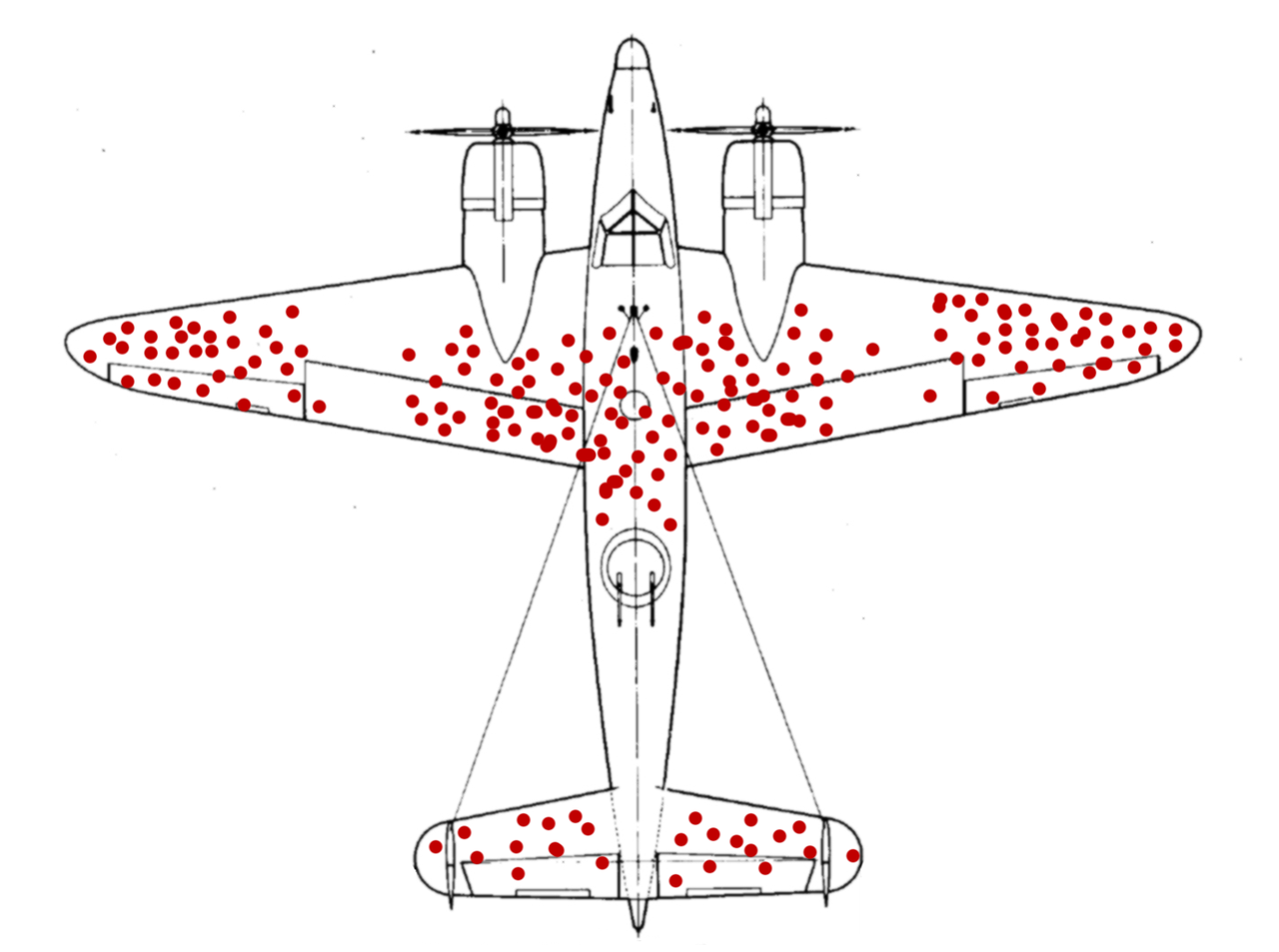
For the inexperience, they probably say, let us add more armor to the wings and the tail wings. Is that correct? The data that was collected is actually from bombers that managed to fly back to base? To gain the right insight, to answer the question, it is best to "query" bombers that are shot down. Yes, now you see it, the data contains survivorship bias. What is survivorship bias?
It is the logical error of concentrating on the people or things that made it past some selection process and overlooking those that did not, typically because of their lack of visibility. This can lead to false conclusions in several different ways. It is a form of selection bias. ~Wikipedia
Abraham Wald was the person who pointed this out, luckily covering that blindspot which can lead to more lives lost, unnecessarily. So Wald proposed that the Navy reinforce areas where the returning aircraft were unscathed since those were the areas that, if hit, would have caused the plane to be struck down.
So is it true that with data, data science can be done? Clearly this example shows otherwise.
Example 2: Income
Most people understand that income drives a lot of different behavior. Let me ask you, are you willing to share exactly how much are you earning? Unless the party asking is from tax services, it is very unlikely that you are going to share the exact amount.
If you are the party collecting the data, you know that it is not a matter of yes or no on collecting income data but rather how to still be able to collect it. You probably am thinking perhaps, I can put income in ranges and let the survey participant choose the appropriate income range. Follow-up question on the range is how shall we set the range then? If the range is too small, survey participants will still not be comfortable sharing their income data. The larger the range, the more information you lose on the income. This means there is a delicate balance here, a Goldilocks moment, too small a range and you lose data points whereas too large a range you lose information on the income data.
So can we take accuracy for granted when it comes to data collection?
Example 3: Staff Turnover Analysis
During a tea break in one of the classes I conducted, a participant sat down to join my table. She began the conversation with a compliment and proceeded to ask me, "Koo, you have a lot of practical knowledge, I hope to go back and apply them in my work. I am looking at doing staff turnover analysis, hoping to understand why staff in my company are leaving. The data that I have for this analysis is the exit interviews I have collected over 10 years. What are your thoughts?"
Let me take this opportunity to ask the readers, go back to the time when you did your last exit interview. What was running in your mind? In my mind, I was thinking, during my last exit interview, "Let's be nice here. There are no benefits for burning bridges here." And I proceed to share that the reason for leaving was anything but the organization's fault (i.e. outside have better opportunities, challenging work scope, etc).
Coming back to the participant's project of analyzing staff turnover, do you think she will get the answers/insights she needs? I highly doubt so given there is a very high likelihood that the data will not reflect the reality accurately.
Through this example you can see, we also need to understand the psychology behind the data collected. We have to ask ourselves what is the possible bias behind the data collected.
Example 4: Forecasting
During my work, I got this question, "Koo, I have my sales data for different categories of goods. I will like to make a forecast now. Is it possible?"
"Can I check how much data do you have and what is the time period you wish to make the forecast?
"I have 3 months of daily sales data, from May to July 20XX. I will like to use the data to forecast the weekly demand a year ahead."
Firstly using 3 months of data to forecast for 12 months ahead is impossible. Why is that so? In forecasting, the models need to detect the following, cycles, seasonality, and trends. To detect them, the models need at least 2 years of data to detect the cycles and the seasons, to make comparisons between years to detect them. And you can see, the more data you can bring to the forecasting models, the better it can do the detection, the more certain the models are of their forecast. Till now the discussion here also includes an important assumption and that is, the organization has data for ALL the time periods. This might not be the case for various reasons like data got corrupted, data was lost, etc.
Simply having data here does not mean you can do meaningful forecasting as see in this example.
Conclusion
We cannot take data collection for granted if we want to gain value from Data Science. Proper planning is needed to ensure that data collected is of the highest quality possible, so that the insights are actionable and relevant.
Thanks for the kind support, taking the time to read till here. If you found this to be useful, consider subscribing to my newsletter. Stay in touch on LinkedIn and Twitter! :)