Recently, there was an article posted at Data Science SG, an interest group that I co-founded. In the article, "Data Science: Reality Doesn't Meet Expectations". Seven (7) common reasons were written on why Data Science initiatives failed. I will be writing my own observations and opinions here, extending from the article. I hope through the articles, your organization can build sustainable data science initiatives and build up the data science capabilities. With the proper data science foundation set, your organization can move on to Artificial Intelligence.
(1) People don’t know what “data science” does
Data science is a very new field. The reason is this is the first time in human history, a lot of us are facing a very large influx of data. Human's familiarity with data stopped at bar charts, pie charts, mean, media, etc. They have not handled data in its entirety, from creation, collection all the way to using it, and strategizing from it.
This is why, not many people understand what data science is and at its face value, people only know that it consists of data and scientific methods use in businesses. Many people are thrust into the field so their impression and understanding of data science are either learned from articles or conversations with friends. There is no guarantee that they understand what data science really is.
In explaining what the current situation with Data Science is, I always like to bring the story up about blind men touching an elephant, a creature that they have not seen before. If you know about the story, you know that each blind men have their version of what an elephant is. Similarly, everyone has their own understanding of what data science is based on what they have read, heard, or discussed.
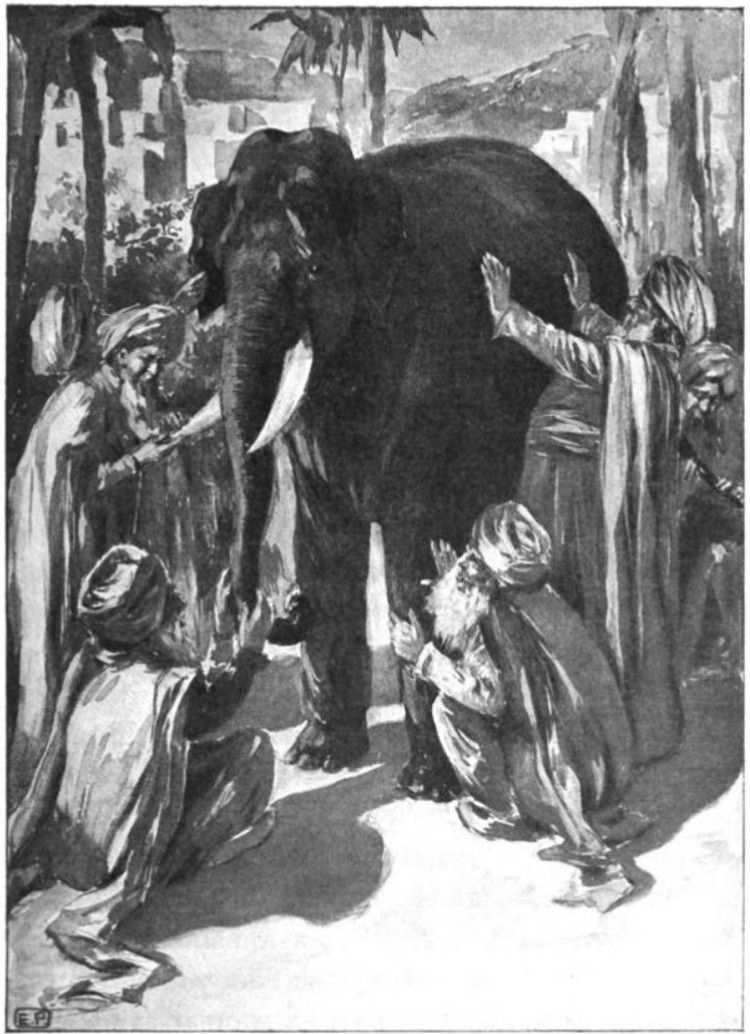
Because of the lack of understanding, communications break down easily when both sides peppered their discussion with "data science" but meaning to each individual is different. It is like one party is thinking about the apple as the fruit and the other party is thinking about Apple as the technology company.
Hiring
And likely some of these people will be entrusted to build up the data science team in their organization. With no plans as to how data science is to be carried out, they are now pushed to hire data scientists. This is where things can collapse very quickly, these managers asked the HR for assistance in setting up the job description (JD) for hire. HR being very new to the field have no idea, decide to copy- &-paste from similar JD, with a VERY STRONG assumption that all data scientists do the same thing, which is NOT TRUE at all.
And during the interview process, with no idea what data science projects will be undertaken, the managers decide to test the breadth of skills, hope to get a data scientist that can do everything which of course is going to be very difficult. And when one is hired, he/she realized the expectation (job description) and reality(work scope) is so much different.
Solution?
The first step is to acknowledge that more understanding is needed. Find a friend who is very experienced in data science and willing to share his/her expertise. If not consider hiring a mentor/consultant that is experienced.
With an experienced data scientist with you, plan out your data science initiatives. Have a read on this post for better ideas. With the roadmap, seek the assistance of the experienced data scientist to hire people for your organization. If possible, have the mentor to sit in during the interviews. Data scientists are like diamonds, it takes one to know one. With the right hire and expectation set, that will be a good foundation to build from for a lasting data science capabilities in your organization.
(2) Data science leadership is sorely lacking
As discussed in (1), data science is a new field so the professionals that are versed in the field are not a lot, to begin with. They rely very much on vendors, who are selling data science tools for a living, to understand better. This gives opportunities to vendors to craft marketing messages to their advantage, further exacerbating any misconception on what data science is about. Together with the pressure from management, requiring that value be shown almost immediately, there is really no time to find out about this vast world of data science, as well as artificial intelligence perhaps.
Moreover, without a good understanding of the field and being the decision-maker, chances are there will be a gap between expectation and execution. This creates frustrations for the rest of the team. Bring the inexperienced to projects that create data products, chances are anything that can go wrong with the project can go wrong for instance, overbudget, milestones are set wrong, milestones not reach in time, story points not set properly resulting in over/underestimating the time required.
The lack of data science leadership can lead to too many challenges, getting wrong tools for with an expensive price tag, data science projects gone wrong are just a few of the challenges. And these challenges can add tremendous costs to businesses that may not be easy to recoup back through data science, due to lack of knowledge and relevant experience.
Solution?
Acknowledge that there are gaps in understanding. Find data scientists who are experienced in them. Again it can be your friends or and external consultant. More important is finding someone with the right experience fit. Seek their opinions and feedback actively in matters that relate to data science. What if decisions need to be made in a few days? Consider talking to a consultant perhaps. Check the experience of the consultant. If possible, have a quick chat. If you feel comfortable with the experience and availability of the consultant, then perhaps considering hire them on a retainer basis. Like this, the business can plug the gaps in knowledge and experience, be able to set the right expectations in projects, in building the infrastructure to support the initiatives and hiring the appropriate talents.
Conclusion
As mentioned, data science is a very new field and each and every one of us has our own understanding of it. This makes communication very challenging, and leadership is about good communication as well. This leads to data science leadership that failed. Realize that experience needs to be gained from the right people. Understand where the experience and knowledge gap are and actively seek out the experienced data scientist, it can be a friend or a consultant. Bridging the gap with someone's experience can save a lot of money in the long run and help your business to quickly move up the data science ladder, building data science capabilities that will help your business last longer!
This is part 1 of the series. The article I mentioned has seven reasons altogether. I will cover the rest in other posts later.
If your business is thinking of engaging an external consultant/mentor. Consider chatting with me after checking out my LinkedIn profile. Or we can keep in touch on Twitter.
If what I have discussed here or any of my articles is of value to you, consider subscribing to my newsletter. Have fun in your Data Science and Artificial Intelligence journey!